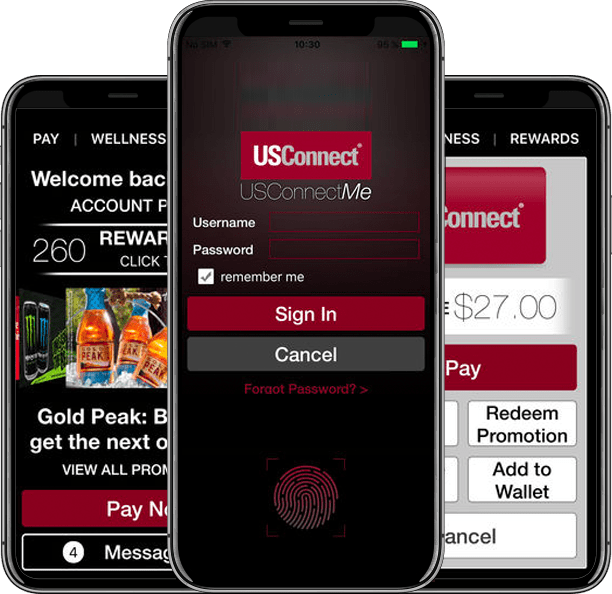
09 Jun How Yami Freshs Vending Machines Boost Productivity
In the rapidly evolving landscape of healthcare, data science has emerged as a transformative force, revolutionizing patient care and diagnosis. By harnessing the power of big data, machine learning, and artificial intelligence, healthcare providers can now deliver more accurate diagnoses, personalized treatments, and improved patient outcomes. This comprehensive guide delves into the myriad ways data science is reshaping healthcare, from predictive analytics to precision medicine, and explores the potential future advancements in this dynamic field.
The Role of Data Science in Healthcare
Data science encompasses a broad range of techniques and tools designed to extract meaningful insights from vast amounts of data. In healthcare, these insights can be used to enhance patient care, streamline operations, and drive innovation. Key areas where data science is making a significant impact include:
- Predictive Analytics: By analyzing historical patient data, predictive models can forecast disease outbreaks, identify high-risk patients, and optimize resource allocation.
- Precision Medicine: Data science enables the development of personalized treatment plans based on an individual’s genetic makeup, lifestyle, and medical history.
- Medical Imaging: Advanced algorithms can analyze medical images to detect abnormalities, assist in diagnosis, and monitor disease progression.
- Clinical Decision Support: Data-driven tools provide healthcare professionals with evidence-based recommendations, improving decision-making and patient outcomes.
- Operational Efficiency: Data science can optimize hospital workflows, reduce wait times, and enhance overall efficiency in healthcare delivery.
Predictive Analytics in Healthcare
Predictive analytics leverages historical data to make informed predictions about future events. In healthcare, this can translate to early disease detection, risk stratification, and proactive intervention. For instance, predictive models can identify patients at high risk of developing chronic conditions such as diabetes or heart disease, allowing for timely preventive measures.
Moreover, predictive analytics can forecast patient admission rates, enabling hospitals to manage resources more effectively. By anticipating patient influx, healthcare facilities can ensure adequate staffing, reduce wait times, and enhance the overall patient experience.
Precision Medicine: Tailoring Treatments to Individuals
Precision medicine is a groundbreaking approach that considers individual variability in genes, environment, and lifestyle when designing treatment plans. By leveraging data science, healthcare providers can develop personalized therapies that are more effective and have fewer side effects.
For example, genomic data can reveal specific genetic mutations that drive cancer growth, allowing oncologists to prescribe targeted therapies. Similarly, pharmacogenomics studies how genes affect a person’s response to drugs, enabling the selection of medications that are most likely to be effective for a particular patient.
Advancements in Medical Imaging
Medical imaging is a critical component of modern healthcare, aiding in the diagnosis and monitoring of various conditions. Data science has significantly enhanced the capabilities of medical imaging through the development of sophisticated algorithms that can analyze images with remarkable accuracy.
Machine learning models can detect subtle patterns in medical images that may be missed by human eyes, leading to earlier and more accurate diagnoses. For instance, deep learning algorithms have demonstrated exceptional performance in identifying tumors, fractures, and other abnormalities in radiographs, MRIs, and CT scans.
Clinical Decision Support Systems
Clinical decision support systems (CDSS) are data-driven tools that assist healthcare professionals in making informed decisions. These systems integrate patient data with evidence-based guidelines to provide recommendations for diagnosis, treatment, and care management.
CDSS can alert clinicians to potential drug interactions, suggest appropriate diagnostic tests, and recommend treatment options based on the latest clinical evidence. By enhancing the decision-making process, CDSS contribute to improved patient outcomes and reduced medical errors.
Enhancing Operational Efficiency
Operational efficiency is crucial for delivering high-quality healthcare in a cost-effective manner. Data science can optimize various aspects of healthcare operations, from scheduling and resource allocation to supply chain management.
For example, predictive analytics can forecast patient demand, enabling hospitals to allocate resources more efficiently. Additionally, data-driven insights can streamline workflows, reduce wait times, and enhance the overall patient experience.
Case Studies: Data Science in Action
Several real-world examples illustrate the transformative impact of data science in healthcare:
- Johns Hopkins Hospital: Implemented a predictive analytics tool to identify patients at risk of sepsis, resulting in a significant reduction in sepsis-related mortality.
- Mayo Clinic: Utilized machine learning algorithms to predict patient outcomes after surgery, enabling personalized postoperative care plans.
- IBM Watson Health: Leveraged natural language processing to analyze medical literature and provide oncologists with evidence-based treatment recommendations.
Future Directions and Challenges
While data science holds immense promise for healthcare, several challenges must be addressed to fully realize its potential:
- Data Privacy and Security: Ensuring the confidentiality and security of patient data is paramount. Robust data protection measures and compliance with regulations such as HIPAA are essential.
- Data Integration: Healthcare data is often fragmented across different systems and formats. Integrating and standardizing this data is crucial for effective analysis.
- Interoperability: Seamless data exchange between healthcare systems is necessary for comprehensive patient care. Interoperability standards and frameworks are needed to facilitate this exchange.
- Ethical Considerations: The use of data science in healthcare raises ethical questions, such as algorithmic bias and the potential for discrimination. Addressing these concerns is vital for equitable and fair healthcare delivery.
Conclusion
Data science is revolutionizing healthcare by enabling more accurate diagnoses, personalized treatments, and improved patient outcomes. From predictive analytics and precision medicine to advanced medical imaging and clinical decision support, the applications of data science are vast and varied. As the field continues to evolve, addressing challenges related to data privacy, integration, and ethics will be crucial for maximizing its potential.
For those interested in exploring the intersection of data science and healthcare further, consider learning more about how Yami Fresh’s vending machines can boost productivity in various settings. By staying informed and embracing innovation, we can collectively work towards a future where data-driven healthcare is the norm, ultimately leading to better patient care and outcomes.